Unlocking AI Efficiency: How MLflow's Gateway Boosts Data Science Workflow
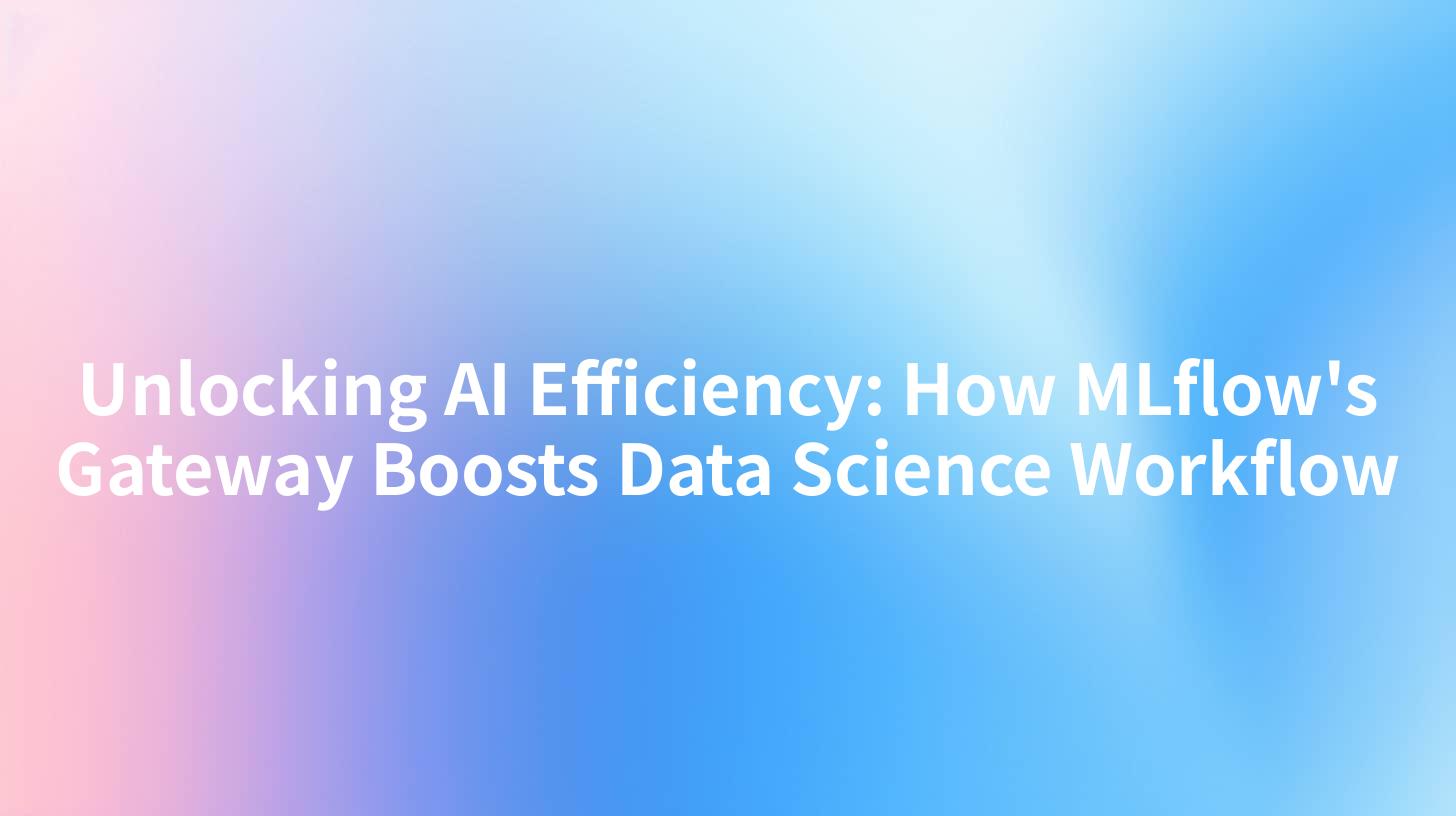
Open-Source AI Gateway & Developer Portal
In the ever-evolving landscape of data science, efficiency and scalability are paramount. The integration of artificial intelligence (AI) into data science workflows has revolutionized the way organizations operate, providing unprecedented insights and decision-making capabilities. One such tool that has gained significant traction in the field is MLflow, an open-source platform designed to manage the ML lifecycle. This article delves into the intricacies of MLflow's gateway and its role in enhancing the data science workflow.
Introduction to MLflow
MLflow is an open-source platform to manage the ML lifecycle, from experimentation to deployment. It allows data scientists to track experiments, compare results, and deploy models. MLflow's core components include MLflow Tracking, MLflow Models, and MLflow Projects. Each of these components plays a crucial role in the data science workflow, but the gateway is perhaps the most pivotal.
The Role of MLflow's Gateway
The MLflow gateway serves as a single entry point for all ML services. It acts as a bridge between the data science team and the production environment, ensuring seamless integration and efficient operation. By serving as a centralized hub for ML services, the gateway simplifies the deployment and management of AI models.
Centralized Management
One of the primary benefits of the MLflow gateway is centralized management. With the gateway, data scientists can manage all their ML services from a single interface. This eliminates the need for multiple tools and platforms, streamlining the workflow and reducing the complexity of managing ML services.
Simplified Deployment
The MLflow gateway simplifies the deployment of AI models by automating the process. It abstracts away the complexities of infrastructure and deployment, allowing data scientists to focus on their core work. This not only saves time but also reduces the likelihood of errors during deployment.
Enhanced Scalability
Scalability is a critical aspect of any data science workflow. The MLflow gateway provides a scalable architecture that can handle the increasing demands of AI models. It ensures that as the volume of data and the complexity of models grow, the system remains robust and efficient.
Improved Collaboration
Collaboration is essential in data science, and the MLflow gateway facilitates it. It allows team members to share their work, collaborate on experiments, and track changes. This fosters a culture of collaboration and ensures that everyone is on the same page.
APIPark is a high-performance AI gateway that allows you to securely access the most comprehensive LLM APIs globally on the APIPark platform, including OpenAI, Anthropic, Mistral, Llama2, Google Gemini, and more.Try APIPark now! πππ
APIPark: Enhancing MLflow's Gateway
While MLflow's gateway is a powerful tool, it can be further enhanced with the integration of other platforms. One such platform is APIPark, an open-source AI gateway and API management platform. APIPark can be integrated with MLflow's gateway to provide additional functionality and improve the overall efficiency of the data science workflow.
Features of APIPark
APIPark offers a range of features that complement MLflow's gateway. Here are some of the key features:
- Quick Integration of 100+ AI Models: APIPark allows for the integration of various AI models with a unified management system for authentication and cost tracking.
- Unified API Format for AI Invocation: It standardizes the request data format across all AI models, ensuring that changes in AI models or prompts do not affect the application or microservices.
- Prompt Encapsulation into REST API: Users can quickly combine AI models with custom prompts to create new APIs, such as sentiment analysis, translation, or data analysis APIs.
- End-to-End API Lifecycle Management: APIPark assists with managing the entire lifecycle of APIs, including design, publication, invocation, and decommission.
- API Service Sharing within Teams: The platform allows for the centralized display of all API services, making it easy for different departments and teams to find and use the required API services.
Integrating APIPark with MLflow
Integrating APIPark with MLflow's gateway is straightforward. Here's a step-by-step guide:
- Install APIPark: Use the following command to install APIPark:
bash curl -sSO https://download.apipark.com/install/quick-start.sh; bash quick-start.sh
- Configure APIPark: Once installed, configure APIPark to work with your MLflow environment.
- Deploy AI Models: Use APIPark to deploy your AI models to the MLflow gateway.
- Monitor and Manage: Use APIPark's management tools to monitor and manage your AI models.
Conclusion
The integration of MLflow's gateway with APIPark provides a robust and efficient platform for managing the data science workflow. By combining the strengths of both platforms, organizations can streamline their AI processes, enhance collaboration, and achieve greater scalability.
FAQs
Q1: What is MLflow's gateway? A1: MLflow's gateway is a single entry point for all ML services, acting as a bridge between the data science team and the production environment.
Q2: How does APIPark enhance MLflow's gateway? A2: APIPark enhances MLflow's gateway by providing additional features such as quick integration of AI models, unified API format, and end-to-end API lifecycle management.
Q3: Can APIPark be integrated with MLflow? A3: Yes, APIPark can be integrated with MLflow to provide additional functionality and improve the overall efficiency of the data science workflow.
Q4: What are the benefits of using APIPark with MLflow? A4: The benefits include centralized management, simplified deployment, enhanced scalability, and improved collaboration.
Q5: How do I install APIPark? A5: You can install APIPark using the following command: bash curl -sSO https://download.apipark.com/install/quick-start.sh; bash quick-start.sh
πYou can securely and efficiently call the OpenAI API on APIPark in just two steps:
Step 1: Deploy the APIPark AI gateway in 5 minutes.
APIPark is developed based on Golang, offering strong product performance and low development and maintenance costs. You can deploy APIPark with a single command line.
curl -sSO https://download.apipark.com/install/quick-start.sh; bash quick-start.sh
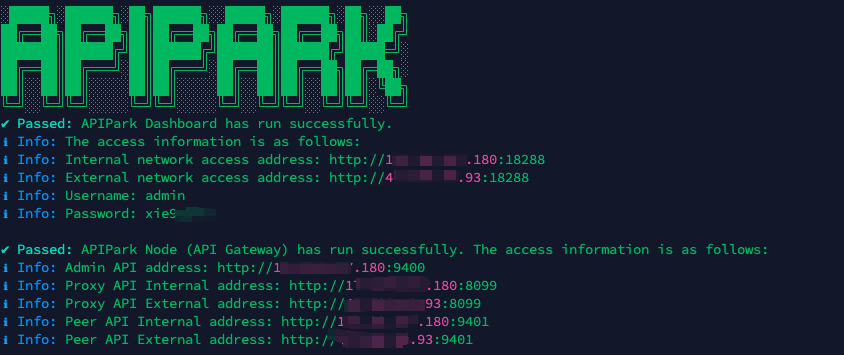
In my experience, you can see the successful deployment interface within 5 to 10 minutes. Then, you can log in to APIPark using your account.
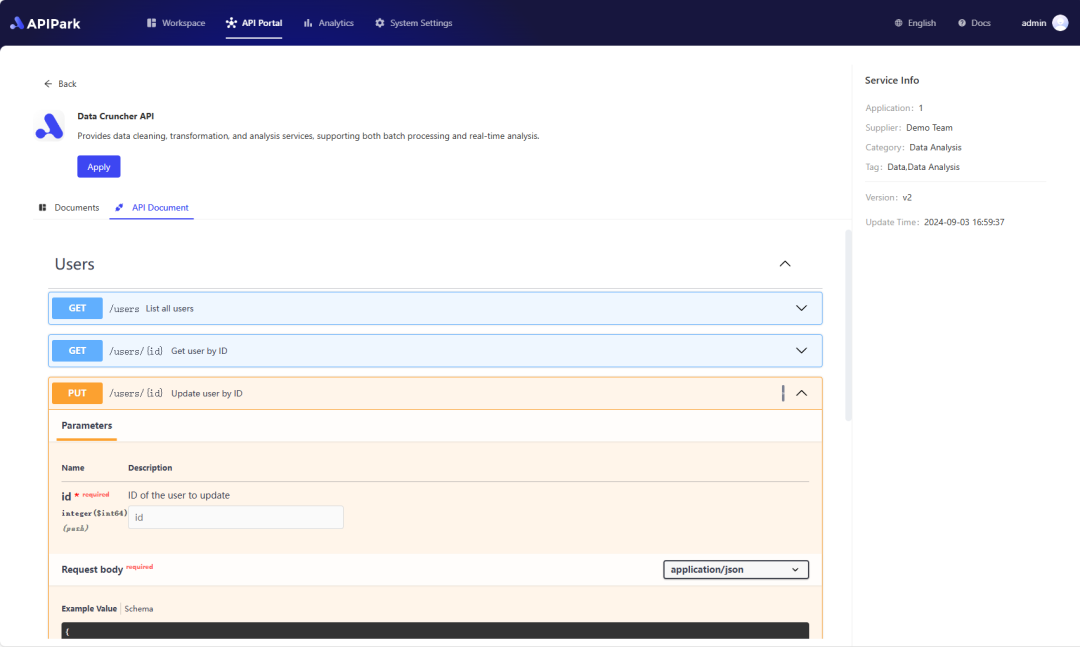
Step 2: Call the OpenAI API.
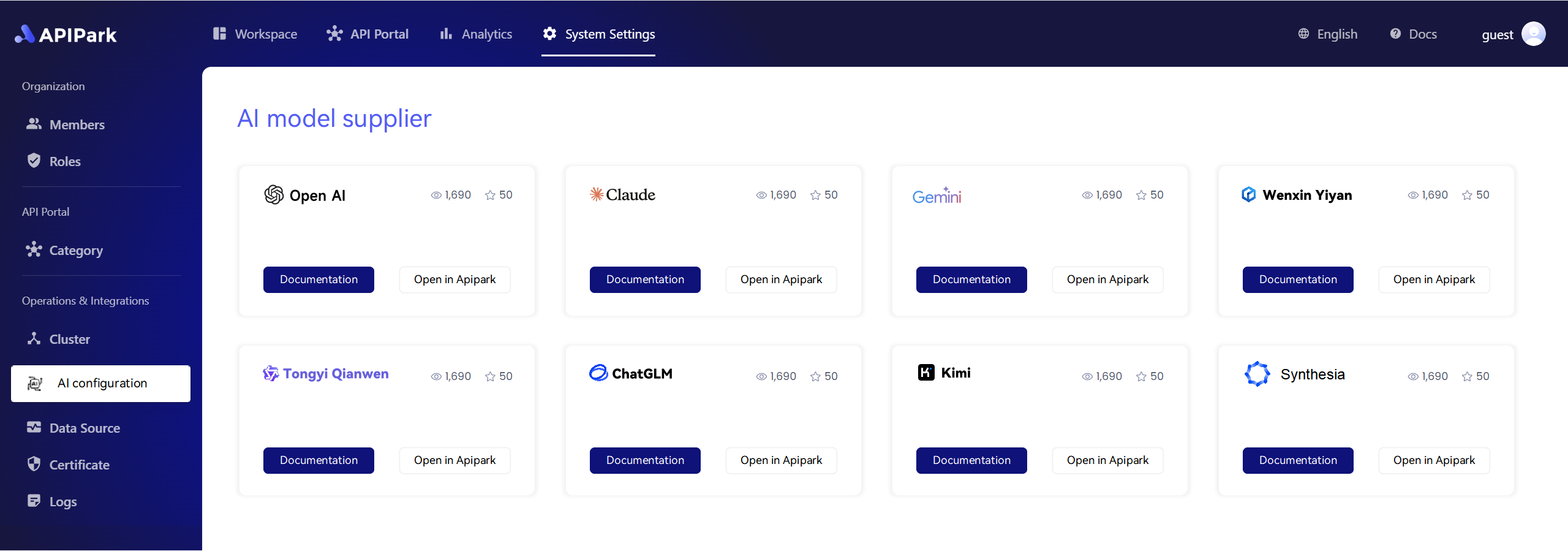